In this section, you can evaluate the effect of climate change on animal species distribution in order to take appropriate measures that help the European Union to conserve biodiversity. For the evaluation of the effect of such changes, the development of robust and generalizable distribution models is considered for biology conservation, which relate the geographical distribution of a species to the multiple decisive Environmental factors, as well as inferring and forecasting their potential distribution in the future from its past condition.
This section describes the implementation of camera traps in recording mammalian presence along Sierra de las Nieves National Park. To enhance the accuracy of localization and classification of mammals in photographs, an automatic artificial intelligence detection procedure has been developed. This new approach will replace the manual labelling process, traditionally used in photo-trapping campaigns.
The cameras have motion sensors, triggered for photo or video shooting when animals pass at short distances from them.
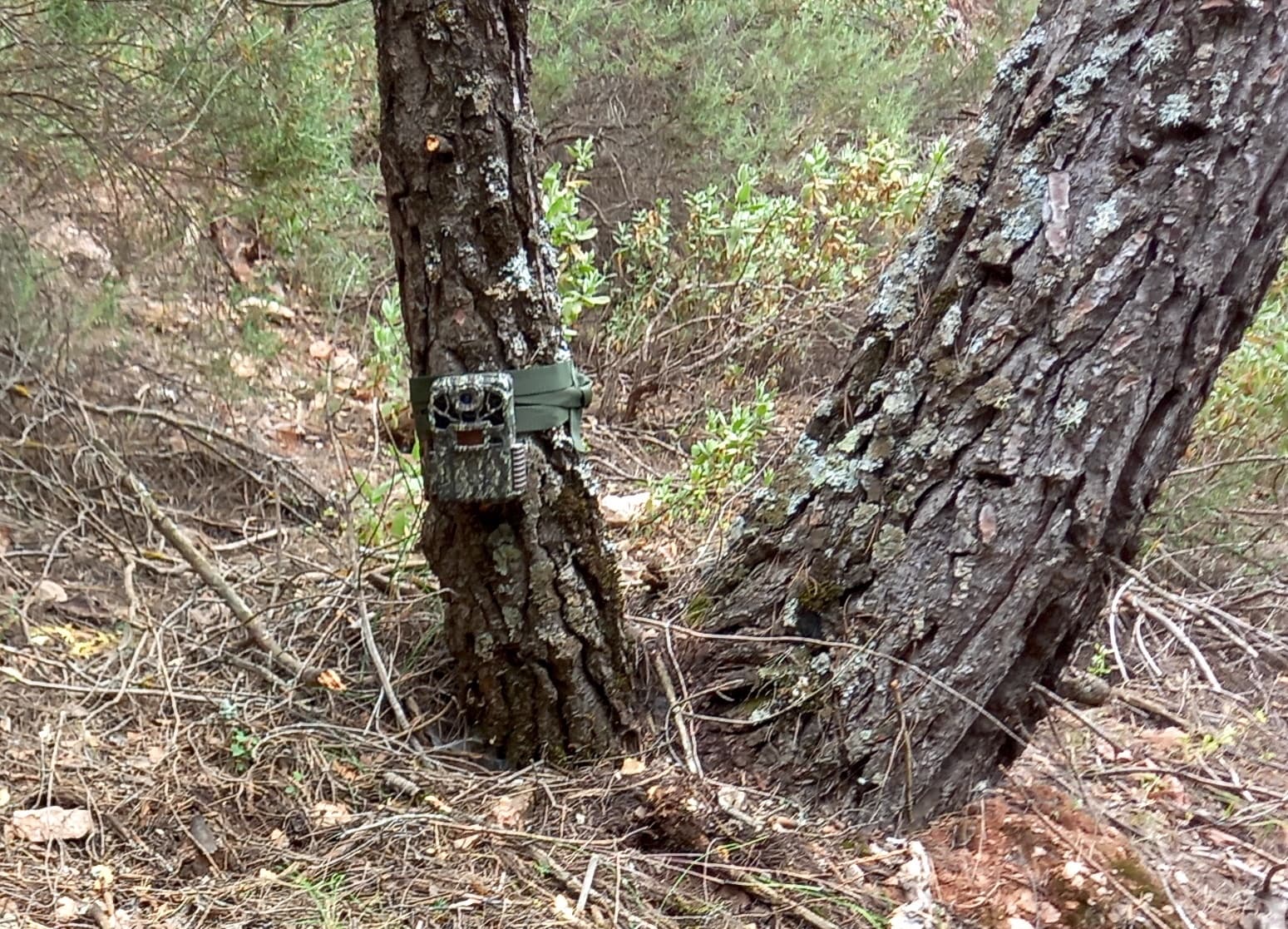
Field work is being carried out in the recently declared Sierra de las Nieves National Park, Málaga, an area of special interest due to its southern location in Europe, which makes it especially vulnerable to the effects of warming and drought associated with climate change. Besides, there is a need to obtain updated data about mammals in the National Park for research and conservation, as given its new highly protected status the information is scarce, particularly for carnivores.
So far, sampling carried out to date have detected the presence of seven species of carnivores: red fox; stone marten; Egyptian mongoose; common genet; Eurasian otter; Eurasian badger and least weasel, and five wild ungulates: red deer; roe deer; Iberian ibex; European mouflon and wild boar. We have also detected the presence of rabbits, some small mammals (rodents and insectivores) and some domestic species such as horse; cow; sheep; goat; dog and cat.
All the data collected by camera traps (images and associated databases) contributes to the Lifewatch ERIC “EnBIC2-Lab” virtual research lab and will also be shared with the National Park authorities for management purposes. The preliminary results of the project include statistical models that help understanding mammals’ spatial distribution and activity patterns:
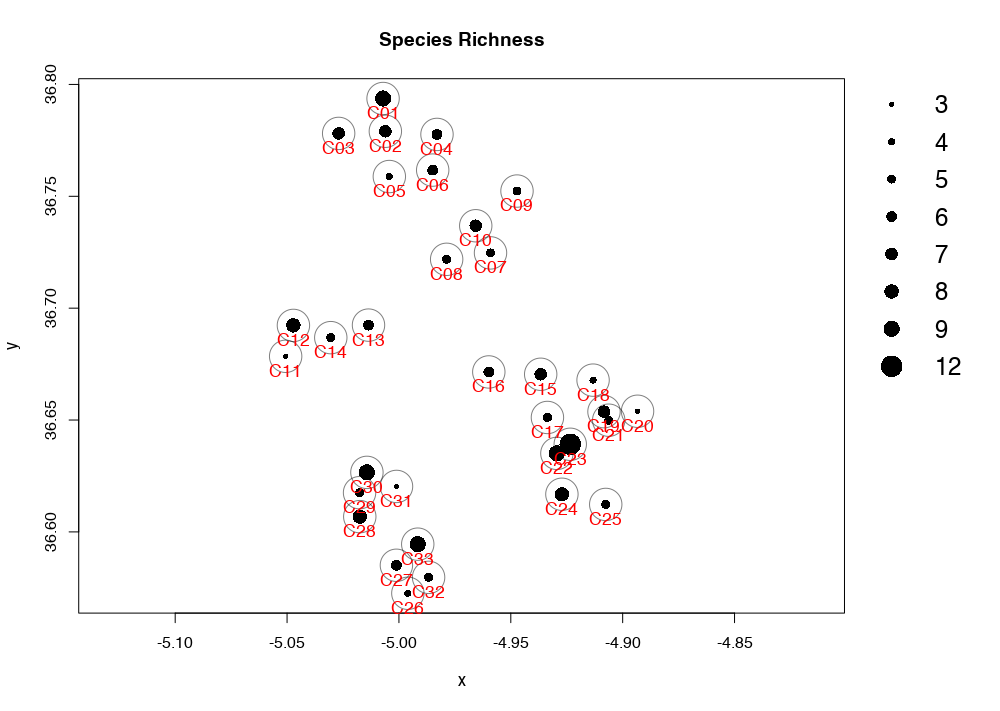
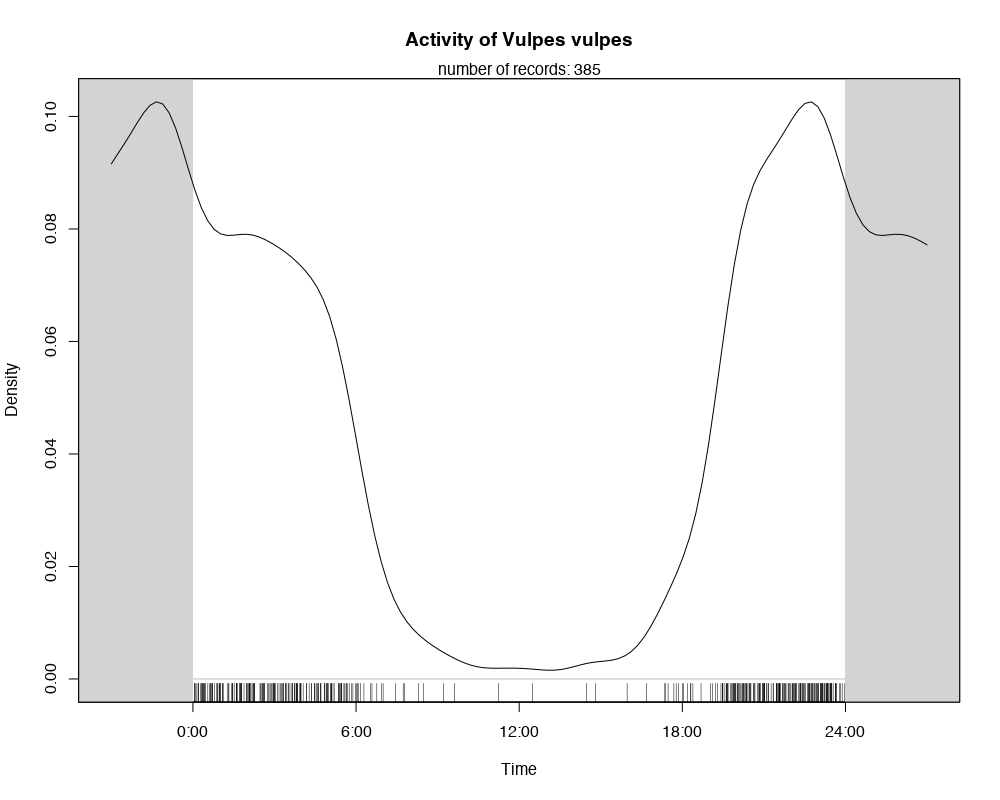
Camera traps produce a high volume of images, which traditionally need to be manually reviewed to identify the species that appear in them. This process is very laborious, so we are currently developing an automatic classification procedure using artificial intelligence.
For this, we have developed a Big Data workflow that processes a large group of images. They have been manually labelled by experts previously, so as to later carry out a model training by means of innovative and artificial intelligence techniques, such as Deep learning algorithms, capable of learning from big amounts of data. The YOLOv4 (You Only Look Once) convolutional algorithm has been used. It allows the automatic detection of animals in the different shots by using the COCO (Common Objects in Context) data set, which contains a huge number of objects images automatically labelled.
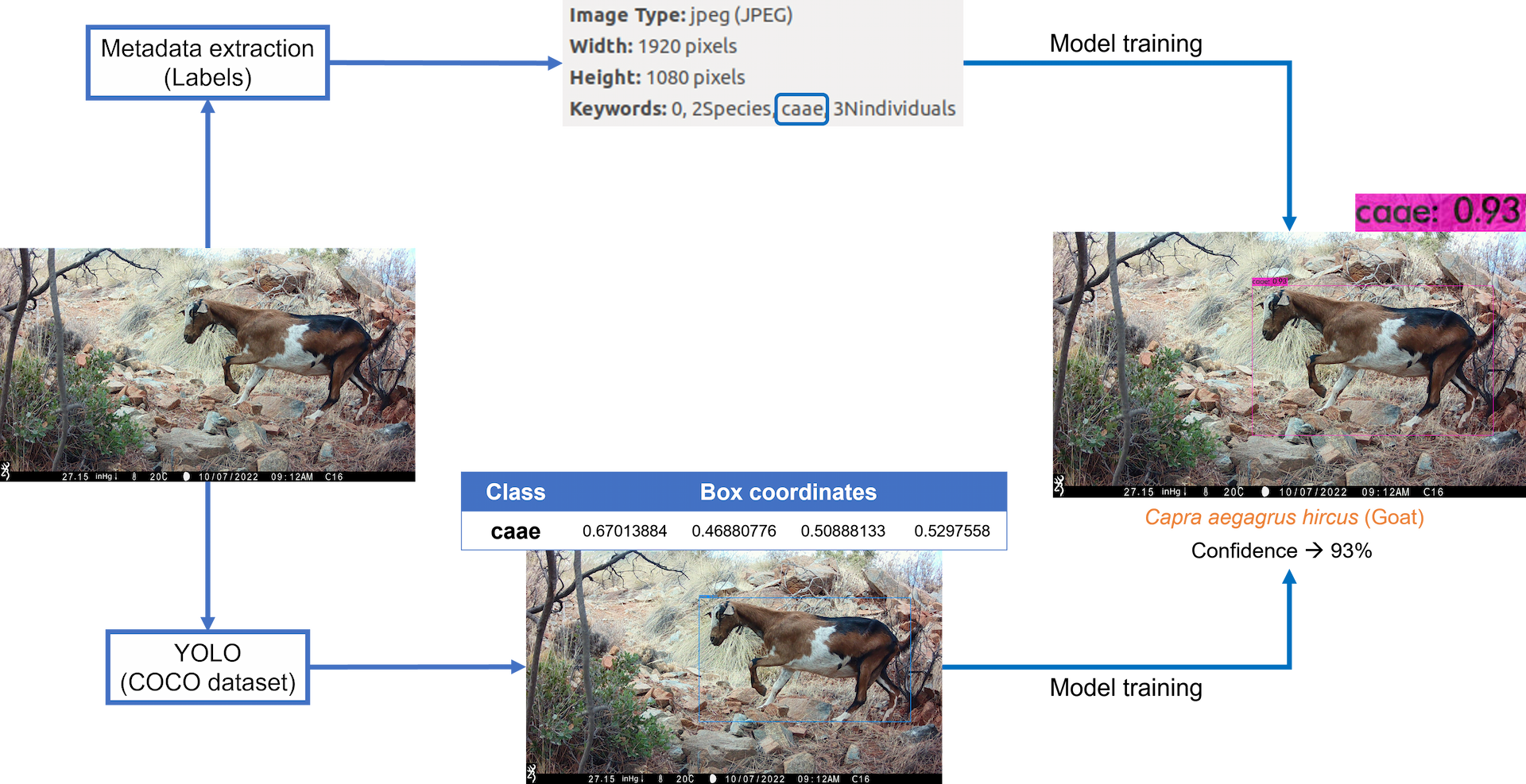
This workflow can be synthesized as follows:
- Automatic animal (object) localization within photo-trapping pictures by taking the COCO data set. As a result, images with the framed animals and their coordinates will be stored.
- Categorization of those framed animals in each picture thanks to the labels contained in their metadata.
Finally, a new set of images has been obtained. In them, every animal has been located, labelled and classified.
To optimize the previous procedure, this has been repeated using more current versions of YOLO, such as YOLOv7, and by paying more attention to animal detection in the pictures, replacing the COCO data set with a new one trained exclusively on animals in each iteration. In addition, training has been carried out with empty images (that is, without animals), also taken from photo-trapping campaigns with the purpose of specializing the detection model, ignoring the picture background.
After carrying out the detection and labelling workflow of every picture, the obtained image set is used to train a deep learning model which will automatically tag new data sets with large amounts of mammal images, without the need to spend hours in manual classifications nor in the labelling on each of the photographs taken from any photo-trapping campaign.